Transforming Business with Advanced Labelling Tool Machine Learning
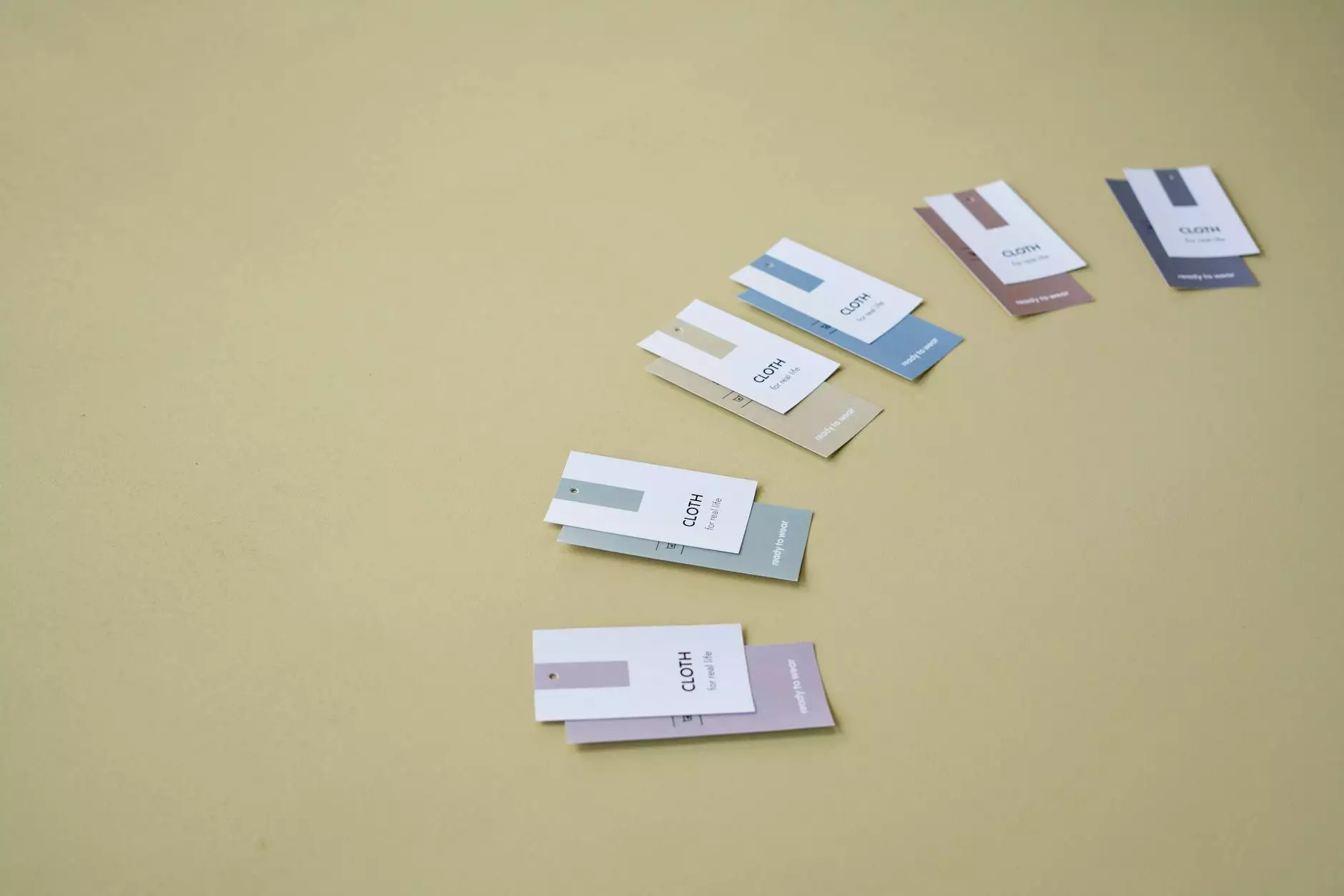
In today’s fast-paced digital landscape, businesses are increasingly reliant on machine learning to optimize operations, enhance customer experiences, and gain competitive advantages. At the heart of successful machine learning projects lies a crucial component: data. More specifically, the quality and quantity of data utilized in training models are imperative for their effectiveness. This is where labeling tools for machine learning become indispensable.
Understanding the Role of Data Annotation
Data annotation involves the process of labeling data to create the necessary groundwork for machine learning models. When data is labeled accurately, it enables predictive analysis and automation, allowing businesses to make informed decisions. Data can be text, images, videos, or audio, and its accurate annotation is essential for various applications including:
- Image recognition
- Natural language processing
- Sentiment analysis
- Object detection in video surveillance
The Impact of Quality Data Annotation Tools
Utilizing high-quality data annotation tools can significantly enhance the efficiency and accuracy of data science projects. Whether you are a startup or an established enterprise, investing in a robust labelling tool machine learning platform can yield tremendous benefits:
- Increased Speed: Automation of labeling processes drastically reduces the time required to prepare datasets.
- Improved Accuracy: Advanced tools minimize human error, leading to better quality data.
- Scalability: Easily adapt your labeling operations as your data requirements grow.
- Collaboration: Facilitate teamwork through cloud-based solutions that allow multiple users to annotate data simultaneously.
Key Features of Effective Labelling Tools
The landscape of machine learning demands tools that do more than just basic data labeling. Here are essential features that a superior data annotation platform should encompass:
1. Versatile Annotation Types
Different projects require various annotation types. High-end labelling tools should support:
- Bounding boxes for object detection
- Polygon annotations for intricate shapes
- Semantic segmentation for deeper understanding of scenes
- Text and voice annotation for NLP applications
2. User-Friendly Interface
A clean and intuitive interface ensures that annotators can work efficiently, reducing the learning curve and expediting the labeling process.
3. Integration Capabilities
The ability to integrate with existing machine learning frameworks like TensorFlow, PyTorch, or other data storage solutions is crucial for seamless workflows. This means that once data is annotated, it can be effortlessly plugged into your ML pipelines.
4. Robust Quality Control
Effective labeling tools include built-in quality control mechanisms, such as:
- Random sampling of data for review
- Consensus models to gauge annotator accuracy
- Feedback loops to continuously improve annotation quality
How Businesses Can Leverage Machine Learning Labelling Tools
Businesses can harness the power of sophisticated labelling tool machine learning solutions through an array of applications:
1. Enhancing Customer Experience
By employing machine learning algorithms that are trained on accurately annotated data, businesses can provide personalized experiences, predict customer preferences, and streamline support operations.
2. Optimizing Marketing Strategies
Data-driven marketing strategies are crucial for targeting and conversion. Businesses can analyze sentiments from various data sources and tailor campaigns that resonate with their audience.
3. Boosting Operational Efficiency
With the integration of machine learning, processes such as inventory management, demand forecasting, and supply chain optimization can be transformed, leading to cost savings and productivity gains.
Choosing the Right Data Annotation Platform
Your success hinges on selecting the right data annotation platform. Here are critical factors to consider:
1. Scalability and Flexibility
As your projects and data requirements evolve, your chosen tool should accommodate growth without requiring complete overhauls.
2. Support and Training
Evaluate the level of customer support and training offered by the platform provider. A responsive support team can significantly reduce downtime and improve your team's efficiency.
3. Cost-Effectiveness
Analyze the pricing structures available and consider your long-term budget. Determine the features that matter most to your business and balance them against costs.
The Future of Data Annotation and Machine Learning
As the field of labelling tool machine learning continues to evolve, advancements such as AI-assisted annotation will streamline processes further, allowing businesses to focus on strategic initiatives rather than manual data entry. With tools becoming ever more sophisticated, companies must stay informed about developments to leverage the latest technology effectively.
Conclusion: Investing in the Future with KeyLabs
A robust data annotation tool is not merely an option; it is a necessity in the modern business ecosystem. By investing in advanced labelling tool machine learning platforms offered by KeyLabs, businesses can ensure they are equipped to tackle the challenges of today’s data-driven world, unleash the full potential of their datasets, and maintain a competitive edge.
Get Started with KeyLabs Today!
Don’t let subpar data hold your business back. Discover how our premier data annotation solutions can catalyze your machine learning efforts. Visit keylabs.ai and take the first step towards transforming your data into actionable insights.